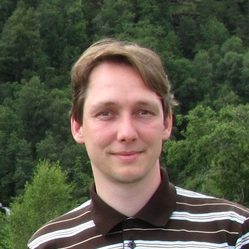
Reik Donner
Magdeburg-Stendal University of Applied Sciences, Potsdam Institute for Climate Impact Research, Potsdam
Title: Towards a better understanding of seasonal climate variability using causal discovery methods
Summary: The Earth’s climate system is characterized by extremely complex dynamics originating from an enormous variety of intrinsically nonlinear physical processes that are acting and interacting at a vast range of temporal and spatial scales. While numerical models nowadays allow skillful weather forecasts for up to 10-14 days in advance, predicting upcoming conditions over lead times of months or even longer requires rather different approaches exploiting knowledge about processes evolving at such longer time scales. Recently, causal discovery has demonstrated to provide versatile tools for improving our understanding of so-called climate teleconnections, which manifest empirical relationships between characteristic spatio-temporal patterns separated by spatial and temporal scales far beyond classical weather regimes that are key for improved seasonal and even longer-term predictions. In my talk, I will discuss some recent advances in the development of such causal discovery methods along with selected successful applications to studying climate variability at seasonal scales in different regions of the Earth.
Reik Donner is a Professor for Data Science and Stochastic Modelling at the Department of Water, Environment, Construction and Safety, Magdeburg-Stendal University of Applied Sciences. Being trained as physicist and mathematician focusing on complex system theory, his main fields of research include nonlinear time series analysis, extreme events and complex networks, along with their application in climate as well as other fields of the Earth and environmental sciences
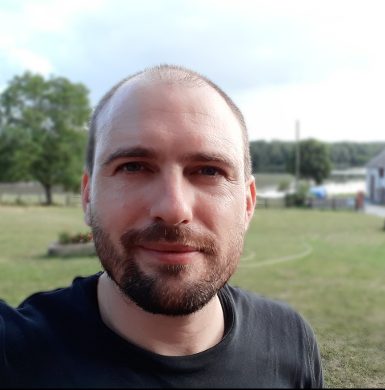
Germain Forestier
IRIMAS, Université de Haute-Alsace
Titre : Apprentissage profond pour la classification de séries temporelles
Résumé : Au cours des dernières années, les approches d’apprentissage profond (deep learning) ont connu un énorme succès dans de multiples domaines tels que le traitement d’images, la vision par ordinateur, la reconnaissance de son et le traitement du langage naturel. Dans cet exposé, je passerai en revue les avancées récentes de l’apprentissage profond pour la classification de séries temporelles univariées et multivariées. Je présenterai des résultats expérimentaux obtenus avec les principales architectures proposées dans la littérature. J’aborderai également les principaux défis liés à l’utilisation de l’apprentissage profond comme l’apprentissage par transfert, l’augmentation de données, la distillation des connaissances, l’interprétabilité et l’apprentissage de représentation dans le contexte de l’analyse des séries temporelles. Je présenterai des applications sur des benchmarks de séries temporelles et dans les domaines de l’analyse d’images satellitaires et de l’analyse du mouvement humain.
Germain Forestier est titulaire d’un doctorat en informatique de l’Université de Strasbourg. Il a passé un an en tant que post-doctorant à l’INRIA Rennes / INSERM, où il a travaillé sur l’analyse de données biomédicales. Il est actuellement Professeur des Universités en informatique à l’Université de Haute-Alsace (France) et occupe également un poste de professeur associé à la Monash University (Australie). Ses domaines de recherche incluent la science des données, la fouille de données, les séries temporelles, l’apprentissage automatique, le big data, l’intelligence artificielle et l’apprentissage profond. Il est également directeur du département informatique de l’institut de recherche IRIMAS de l’Université de Haute-Alsace.
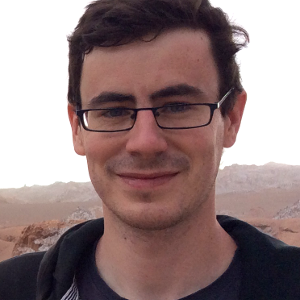
Aidan Hogan
Universidad de Chile, Santiago
Title: Knowledge Graphs in the age of Large Language Models
Summary: The public release of ChatGPT in November 2022 has led to the widespread popularisation and discussion of Large Language Models. Their fame (or infamy) is in large part due to the fact that not only do they encode a vast volume of information, they can also mimic our human language in unprecedented ways. These models thus call into question a fundamental tenet of Data Management: that in order to address non-trivial information needs, the first step is to explicitly structure data in order to lift them from the ambiguous swamp of our human language. This talk will address a pressing question that arises in this context: do we
still need Knowledge Graphs in the age of Large Language Models? We will look at the current capabilities and limitations of Large Language Models, and contrast them with those of Knowledge Graphs. Ultimately we will propose that in many ways, Large Language Models and Knowledge Graphs can be considered complementary, with interleaving strengths and weaknesses. More specifically, we will argue that Large Language Models can help bring the power of Knowledge Graphs to the masses, and the power of the masses to Knowledge Graphs.
Aidan is a Professor and Vice-Director of the Department of Computer Science, University of Chile, and Vice-Director of the Millennium Institute for Foundational Research on Data (IMFD). His research interests primarily relate to the Semantic Web, Graph Databases, Information Extraction and Reasoning; he has published over one hundred peer-reviewed works on these topics. He is an Editor-in-Chief and Co-Founder of the upcoming journal Transactions on Graph Data & Knowledge (TGDK). He is the (co)author of three books, namely « Reasoning on the Web of Data », « The Web of Data », and « Knowledge Graphs ».
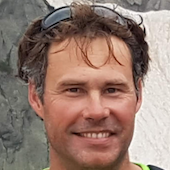
Alexis Joly
INRIA ZENITH, Montpellier
Title : From Pl@ntNet to GeoPl@ntNet: new AI-based approaches for monitoring plant biodiversity
Summary: Pl@ntNet is a citizen science platform that relies on artificial intelligence (AI) to facilitate the identification and inventory of plant species. It is based on a cooperative learning principle: users generate a large number of observations of plants in the field, which are automatically identified by an artificial intelligence algorithm and revised by the community itself, with a weighting principle based on the user’s expertise. Observations that reach a sufficient degree of confidence are then added to the training set of the AI model, which is enriched and progresses over time. In addition, the data produced is used to model species distribution and map biodiversity indicators at very high spatial resolution. To this end, we are working on a new type of model called Deep Species Distribution Models (DeedSDM), which combine a wide range of sources of information on biotic and abiotic factors. These models, trained on large-scale, heterogeneous data, are very promising, but also pose a number of challenges in terms of multi-modal representation, input data bias and prediction uncertainty. Their integration into an interactive cartographic tool, called GeoPlantNet, will help make decisions on species conservation and land use planning.
Alexis Joly is a senior computer scientist with interests in machine learning applied to biodiversity. He is the scientific leader of the Pl@ntNet citizen science project which develops a million-users platform based on AI. He is the founder of the LifeCLEF international virtual lab dedicated to the computer-assisted identification of living organisms.
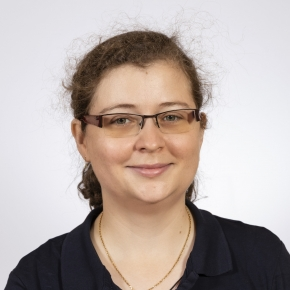
Anne-Cécile Orgerie
IRISA CNRS, Myriads Rennes
Titre: Mesurer et réduire la consommation des systèmes distribués
Résumé : Les systèmes distribués, tels que les clouds, s’étendent de plus en plus à l’échelle mondiale, avec des services numériques hébergés aux quatre coins du globe et appartenant souvent à des systèmes complexes, utilisant eux-mêmes de nombreux autres services et ressources matérielles. Cette augmentation s’accompagne d’une croissance alarmante des infrastructures de cloud et de leur consommation d’énergie. Malgré la complexité des systèmes distribués, il est important de comprendre comment ils consomment l’énergie afin de chasser les Joules gaspillées et de réduire leur impact environnemental. Cet exposé traitera de la mesure de la consommation énergétique des systèmes distribués et de la dérivation de modèles à partir de ces mesures pour évaluer leur consommation énergétique et leur impact environnemental.
Anne-Cécile Orgerie a obtenu son doctorat en informatique en 2011 à Lyon et son habilitation à diriger des recherches en 2020 à Rennes. Depuis 2012, elle est chercheuse au CNRS à l’IRISA à Rennes et fait partie de l’équipe Myriads qui étudie les systèmes distribués à large échelle, le Cloud et le Edge computing. Ses thématiques de recherches portent sur la mesure, la modélisation, la simulation et la réduction de la consommation énergétique des systèmes distribués. Elle est actuellement directrice du groupement de service du CNRS EcoInfo qui étudie les impacts environnementaux du numérique.